Registration Dossier
Registration Dossier
Data platform availability banner - registered substances factsheets
Please be aware that this old REACH registration data factsheet is no longer maintained; it remains frozen as of 19th May 2023.
The new ECHA CHEM database has been released by ECHA, and it now contains all REACH registration data. There are more details on the transition of ECHA's published data to ECHA CHEM here.
Diss Factsheets
Use of this information is subject to copyright laws and may require the permission of the owner of the information, as described in the ECHA Legal Notice.
EC number: 680-102-5 | CAS number: 638-51-7
- Life Cycle description
- Uses advised against
- Endpoint summary
- Appearance / physical state / colour
- Melting point / freezing point
- Boiling point
- Density
- Particle size distribution (Granulometry)
- Vapour pressure
- Partition coefficient
- Water solubility
- Solubility in organic solvents / fat solubility
- Surface tension
- Flash point
- Auto flammability
- Flammability
- Explosiveness
- Oxidising properties
- Oxidation reduction potential
- Stability in organic solvents and identity of relevant degradation products
- Storage stability and reactivity towards container material
- Stability: thermal, sunlight, metals
- pH
- Dissociation constant
- Viscosity
- Additional physico-chemical information
- Additional physico-chemical properties of nanomaterials
- Nanomaterial agglomeration / aggregation
- Nanomaterial crystalline phase
- Nanomaterial crystallite and grain size
- Nanomaterial aspect ratio / shape
- Nanomaterial specific surface area
- Nanomaterial Zeta potential
- Nanomaterial surface chemistry
- Nanomaterial dustiness
- Nanomaterial porosity
- Nanomaterial pour density
- Nanomaterial photocatalytic activity
- Nanomaterial radical formation potential
- Nanomaterial catalytic activity
- Endpoint summary
- Stability
- Biodegradation
- Bioaccumulation
- Transport and distribution
- Environmental data
- Additional information on environmental fate and behaviour
- Ecotoxicological Summary
- Aquatic toxicity
- Endpoint summary
- Short-term toxicity to fish
- Long-term toxicity to fish
- Short-term toxicity to aquatic invertebrates
- Long-term toxicity to aquatic invertebrates
- Toxicity to aquatic algae and cyanobacteria
- Toxicity to aquatic plants other than algae
- Toxicity to microorganisms
- Endocrine disrupter testing in aquatic vertebrates – in vivo
- Toxicity to other aquatic organisms
- Sediment toxicity
- Terrestrial toxicity
- Biological effects monitoring
- Biotransformation and kinetics
- Additional ecotoxological information
- Toxicological Summary
- Toxicokinetics, metabolism and distribution
- Acute Toxicity
- Irritation / corrosion
- Sensitisation
- Repeated dose toxicity
- Genetic toxicity
- Carcinogenicity
- Toxicity to reproduction
- Specific investigations
- Exposure related observations in humans
- Toxic effects on livestock and pets
- Additional toxicological data

Biodegradation in water: screening tests
Administrative data
- Endpoint:
- biodegradation in water: ready biodegradability
- Type of information:
- (Q)SAR
- Adequacy of study:
- key study
- Study period:
- 15/10/2020
- Reliability:
- 2 (reliable with restrictions)
- Rationale for reliability incl. deficiencies:
- accepted calculation method
- Justification for type of information:
- 1. SOFTWARE
QSAR
2. MODEL (incl. version number) : Model 4.0.4 Molcode Ltd., Turu 2, Tartu, 51014, Estonia; http://www.molcode.com
Endpoint (OECD Principle 1): QSAR 2.3.a
Algorythm (OECD Principle 2): QSAR ANN model for Persistence: Biotic degradation in water, Model version 22.05.2012
3. SMILES OR OTHER IDENTIFIERS USED AS INPUT FOR THE MODEL
SMILES: CCCCCCON=O, not used for prediction
3D Mol file used for prediction
4. SCIENTIFIC VALIDITY OF THE (Q)SAR MODEL
[Explain how the model fulfils the OECD principles for (Q)SAR model validation. Consider attaching the QMRF or providing a link]
- Defined endpoint: QSAR 2.3.a; Ready Biodegradability (Aerobic Mineralisation in Surface Water - Simulation Biodegradation Test); OECD 309
- Unambiguous algorithm: QSAR ANN model for Persistence: Biotic degradation in water (Reference to QMRF - attached separately)
- Defined domain of applicability:
a) descriptor domain
b) structural fragment domain
c) mechanims domain
- Uncertainty of the prediction (OECD Principle 4):
The source experimental data for the model originate from different labs and different experiment series, adding to uncertainty, however, previous (and present) successful modeling add to the consi stence of the data. The significant size of the dataset; the diversity of the structures covering a large parts of the chemical space, and the statistical quality (RMS, correlation coefficients etc.) of the model supports reliable predictions within the margins of the experimental error. The similarity of the analogues together with the correct estimates supports potential prediction consistency.
Considering the dataset size, model statistical quality and prediction reliability, a reliability score (Klimisch score) “2” could be assigned to the present prediction.
The prediction reliability in terms of the persistence category is estimated as 84 %
- Mechanistic interpretation:
Chemical degradation half-life has been defined as a quasi-intensive property since it is independent of quantity and is a characteristic of a molecule within a defined environmental medium. The struc tural features of a chemical determine its intrinsic ability or tendency to persist in the environment, independent of its partitioning properties and environmental conditions. Particular features accepted as indicators of high persistence include (per)halogenated aliphatic and aromatic carbon systems, the molecular size being also important.
Because of the nonlinear nature of the ANNs, deeper analysis of the descriptors is difficult compared to the normal multilinear analysis. The present ANN model descriptors are mainly related to the c harge distributions of the compounds e.g. FPSA3 Fractional PPSA (PPSA-3/TMSA) (Zefirov), Negatively Charged Surface Area (Zefirov), Partial Charged (Zefirov) Surface Area of H atoms. In additio n the halogens reactivity plays also important role for the water degradation. Generally the halogens have larger LogT values, the tendency that well agrees with the general understanding of the pro perty.
Artificial Neural networks (ANN) are used in many areas, such as pattern recognition, process analysis and non-linear modelling. An advantage of neural nets is that the neural net model is very flexi ble in contrast to the classical statistical models. A significant disadvantage is the amount of data needed and the causal ambiguity of the network. The neural net ‘learns’ from examples by one of tw o different approaches, supervised or unsupervised learning. During supervised learning, the system is forced to assign each object in the training set to a specific class, while during unsupervised l earning, the clusters are formed without any prior information. One approach commonly used is multi-layer feed-forward (MLF) networks consisting of three or more layers: one input layer, one outp ut layer and one or more intermediate (hidden) layer (Smiths et al., 1994; Xu et al., 1994; De Saint Laumer et al., 1991).
In this model report: nonlinear regression QSAR artificial neural networks model with architecture 6-5-1 trained with back propagation of the error.
ANN is mentioned many times in REACH related official documents ANN is considered as an acceptable algorithm for non-linear correlations.
5. APPLICABILITY DOMAIN
- Descriptor domain: All descriptor values for Hexyl nitrite fall in the applicability domain (training set value ±30%).
- Structural domain: Hexyl nitrite is structurally similar to the model compounds, the model contains compounds featuring short alkyl chains and ether functionalities The training set contains comp ounds of si milar size to the studied molecule.
- Mechanistic domain: Hexyl nitrite is considered to be in the same mechanistic domain as the molecules in the training set as it is structurally similar to the model compounds.
- Similarity with analogues in the training set: The experimental acute oral toxicity values for compounds of similar functionalities (saturated alkyl ethers) all fall to the “low persistence” category, The structural analogues are relatively similar to the studied compound. The descriptor values of the analogues are close to those of the studied compound. The analogues are considered to be within the same mechanistic domain. All the analogues are relativ ely well estimated within the model. The following aspects have been considered for the selection and analysis of structural analogues:
Presence and number of common functional groups;
Presence and relevance of non-common functional groups;
Similarity of the ‘core structure’ apart from the (non-)common functional groups;
Potential differences due to reactivity;
Potential differences due to steric hindrance;
Presence of structural alerts;
Position of the double bonds;
Presence of stereoisomers.
6. ADEQUACY OF THE RESULT
6.1 Regulatory purpose:
The present prediction may be used for preparing the REACH Joint Registration Dossier on the Substance(s) for submission to the European Chemicals Agency (“ECHA”) as required by Regulation (EC) N° 1907/2006 of the European Parliament and of the Council of 18 December 2006 concerning the Registration, Evaluation, Authorisation and Restriction of Chemicals ("REAC H") and as required by Biocide Product Directive 98/8/EC ("98/8/EC")
6.2 Approach for regulatory interpretation of the model result
The predicted result has been presented in the formats directly usable for the intended regulatory purposes. The predicted numeric value in physicochemical units has been presented. The possible classification of the values according to classification systems has also been discussed.
6.3 Outcome
See section 3.2(e) for the classification of the prediction in light of the regulatory purpose described in 4.1.
6.4 Conclusion
Considering the above, the predicted result can be considered adequate for the regulatory conclusion described in 4.1.
Data source
Reference
- Reference Type:
- study report
- Title:
- Unnamed
- Year:
- 2 020
- Report date:
- 2020
Materials and methods
Test guidelineopen allclose all
- Qualifier:
- according to guideline
- Guideline:
- other: OECD Guideline 309: Ready Biodegradability (Aerobic Mineralisation in Surface Water - Simulation Biodegradation Test)
- Deviations:
- no
- Qualifier:
- according to guideline
- Guideline:
- OECD Guideline 301 A (Ready Biodegradability: DOC Die Away Test)
- Principles of method if other than guideline:
- 3.3. Comment on endpoint:
The half-life is the time required for the concentration of a substance to halve its original value in a particular environmental medium. The half-lives of organic compounds are among the most commonly used criteria for studying persistence [1]. The semiquantitative data based on expert judgment and actual experimental values have already been suggested by Webster et al. [2] as preferable for half life identification, and are commonly used to develop the widely applied multimedia models [3,4]. In addition, a simple QSPR regression model has been demonstrated to be an useful tool for the identification and prioritization of existing or not yet synthesized potential persistent organic pollutants
3.4.Endpoint units:
The half-life values (in h) were transformed into logarhitmic form
for modelling
3.5.Dependent variable:
log T(0.5)
3.6.Experimental protocol:
The dataset of structurally heterogeneous and highly
representative of many classes of already defined problematic chemicals
includes 206 organic compounds of known half-lives for transformation
into air [6].
3.7.Endpoint data quality and variability:
min value training set: 1.23
max value training set: 4.74
avrg training set: 2.63 - GLP compliance:
- no
Test material
Reference
- Name:
- Unnamed
- Type:
- Constituent
- Test material form:
- liquid
Study design
- Oxygen conditions:
- aerobic
- Inoculum or test system:
- natural water: marine
Parameter followed for biodegradation estimation
- Parameter followed for biodegradation estimation:
- not specified
Results and discussion
% Degradation
- Key result
- Parameter:
- half-life in days (QSAR/QSPR)
- Value:
- > 60
- Remarks on result:
- not readily biodegradable based on QSAR/QSPR prediction
- Details on results:
- A substance fulfils the persistence/vP criterion (P/vP) if the half-life in marine water is higher than 60 days, OR the half-life in fresh- or estuarine water is higher than 40/60 days.
Applicant's summary and conclusion
- Validity criteria fulfilled:
- not applicable
- Interpretation of results:
- under test conditions no biodegradation observed
- Conclusions:
- Hexyl nitrite falls into the "Low persistence" category.
- Executive summary:
Hexyl nitrite falls into the "Low persistence" category.
Information on Registered Substances comes from registration dossiers which have been assigned a registration number. The assignment of a registration number does however not guarantee that the information in the dossier is correct or that the dossier is compliant with Regulation (EC) No 1907/2006 (the REACH Regulation). This information has not been reviewed or verified by the Agency or any other authority. The content is subject to change without prior notice.
Reproduction or further distribution of this information may be subject to copyright protection. Use of the information without obtaining the permission from the owner(s) of the respective information might violate the rights of the owner.
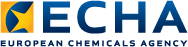